Application of artificial intelligence in various liver and pancreas diseases
-
摘要:
利用人工智能提取出临床血液学数据、影像学图片等大量信息,形成各种可量化的特征,进而分析不同特征与所关心问题(如诊断)的关系,从而解决复杂的医学问题。主要从胰腺癌、肝纤维化及食管静脉曲张入手,阐述并分析了各种人工智能算法分别对上述疾病的诊断效能,以供临床医生更清楚地认识和决策。
Abstract:A large amount of information,such as clinical hematological data and imaging images,can be extracted by artificial intelligence to form various quantifiable features,analyze the association between different features and problems concerned( such as diagnosis),and thus solve complex medical problems. This article elaborates on the efficiency of various artificial intelligence algorithms in the diagnosis of pancreatic cancer,hepatic fibrosis,and esophageal varices,so as to help clinicians with clearer understanding and better decision-making.
-
Key words:
- artificial intelligence /
- pancreatic neoplasms /
- liver cirrhosis /
- esophageal varices /
- diagnosis
-
[1] LE BERRE C,SANDBORN WJ,ARIDHI S,et al. Application of Artificial Intelligence to Gastroenterology and Hepatology[J]. Gastroenterology,2020,158(1):76-94. e2. [2] LECUN Y,BENGIO Y,HINTON G. Deep learning[J]. Nature,2015,521(7553):436-444. [3] FRITSCHER-RAVENS A,BRAND L,KNOFEL WT,et al. Comparison of endoscopic ultrasound-guided fine needle aspiration for focal pancreatic lesions in patients with normal parenchyma and chronic pancreatitis[J]. Am J Gastroenterol,2002,97(11):2768-2775. [4] CASTELLANO G,BONILHA L,LI LM,et al. Texture analysis of medical images[J]. Clin Radiol,2004,59(12):1061-1069. [5] DAS A,NGUYEN CC,LI F,et al. Digital image analysis of EUS images accurately differentiates pancreatic cancer from chronic pancreatitis and normal tissue[J]. Gastrointest Endosc,2008,67(6):861-867. [6] ZHU M,XU C,YU J,et al. Differentiation of pancreatic cancer and chronic pancreatitis using computer-aided diagnosis of endoscopic ultrasound(EUS)images:A diagnostic test[J]. PLo S One,2013,8(5):e63820. [7] OZKAN M,CAKIROGLU M,KOCAMAN O,et al. Age-based computer-aided diagnosis approach for pancreatic cancer on endoscopic ultrasound images[J]. Endosc Ultrasound,2016,5(2):101-107. [8] SFTOIU A,VILMANN P,GORUNESCU F,et al. Efficacy of an artificial neural network-based approach to endoscopic ultrasound elastography in diagnosis of focal pancreatic masses[J]. Clin Gastroenterol Hepatol,2012,10(1):84-90. e1. [9] SFTOIU A,VILMANN P,DIETRICH CF,et al. Quantitative contrast-enhanced harmonic EUS in differential diagnosis of focal pancreatic masses(with videos)[J]. Gastrointest Endosc,2015,82(1):59-69. [10] YANG Y,CHEN H,WANG D,et al. Diagnosis of pancreatic carcinoma based on combined measurement of multiple serum tumor markers using artificial neural network analysis[J]. Chin Med J(Engl),2014,127(10):1891-1896. [11] LIU SL,LI S,GUO YT,et al. Establishment and application of an artificial intelligence diagnosis system for pancreatic cancer with a faster region-based convolutional neural network[J].Chin Med J(Engl),2019,132(23):2795-2803. [12] LI S,JIANG H,WANG Z,et al. An effective computer aided diagnosis model for pancreas cancer on PET/CT images[J].Comput Methods Programs Biomed,2018,165:205-214. [13] GAO X,WANG X. Performance of deep learning for differentiating pancreatic diseases on contrast-enhanced magnetic resonance imaging:A preliminary study[J]. Diagn Interv Imaging,2020,101(2):91-100. [14] DIEHL AM,DAY C. Cause,pathogenesis,and treatment of nonalcoholic steatohepatitis[J]. N Engl J Med,2017,377(21):2063-2072. [15] PISCAGLIA F,CUCCHETTI A,BENLLOCH S,et al. Prediction of significant fibrosis in hepatitis C virus infected liver transplant recipients by artificial neural network analysis of clinical factors[J]. Eur J Gastroenterol Hepatol,2006,18(12):1255-1261. [16] HASHEM S,ESMAT G,ELAKEL W,et al. Comparison of machine learning approaches for prediction of advanced liver fibrosis in chronic hepatitis C patients[J]. IEEE/ACM Trans Comput Biol Bioinform,2018,15(3):861-868. [17] WANG D,WANG Q,SHAN F,et al. Identification of the risk for liver fibrosis on CHB patients using an artificial neural network based on routine and serum markers[J]. BMC Infect Dis,2010,10:251. [18] WEI R,WANG J,WANG X,et al. Clinical prediction of HBV and HCV related hepatic fibrosis using machine learning[J].EBio Medicine,2018,35:124-132. [19] RAOUFY MR,VAHDANI P,ALAVIAN SM,et al. A novel method for diagnosing cirrhosis in patients with chronic hepatitis B:Artificial neural network approach[J]. J Med Syst,2011,35(1):121-126. [20] CHEN Y,LUO Y,HUANG W,et al. Machine-learning-based classification of real-time tissue elastography for hepatic fibrosis in patients with chronic hepatitis B[J]. Comput Biol Med,2017,89:18-23. [21] WANG K,LU X,ZHOU H,et al. Deep learning Radiomics of shear wave elastography significantly improved diagnostic performance for assessing liver fibrosis in chronic hepatitis B:A prospective multicentre study[J]. Gut,2019,68(4):729-741. [22] YASAKA K,AKAI H,KUNIMATSU A,et al. Liver Fibrosis:Deep convolutional neural network for staging by using gadoxetic acid-enhanced hepatobiliary phase MR images[J]. Radiology,2018,287(1):146-155. [23] YASAKA K,AKAI H,KUNIMATSU A,et al. Deep learning for staging liver fibrosis on CT:A pilot study[J]. Eur Radiol,2018,28(11):4578-4585. [24] SOWA JP,ATMACAKAHRAMAN A,et al. Non-invasive separation of alcoholic and non-alcoholic liver disease with predictive modeling[J]. PLo S One,2014,9(7):e101444. [25] ESLAM M,NEWSOME PN,SARIN SK,et al. A new definition for metabolic dysfunction-associated fatty liver disease:An international expert consensus statement[J]. J Hepatol,2020,73(1):202-209. [26] ESLAM M,SANYAL AJ,GEORGE J,et al. MAFLD:A consensus-driven proposed nomenclature for metabolic associated fatty liver disease[J]. Gastroenterology,2020,158(7):1999-2014. e1. [27] GAO X. New thoughts about renaming nonalcoholic fatty liver disease[J]. J Clin Hepatol,2020,36(6):1201-1204.(in Chinese)高鑫.非酒精性脂肪性肝病更名带来的新思考[J].临床肝胆病杂志,2020,36(6):1201-1204. [28] SOWA JP,HEIDER D,BECHMANN LP,et al. Novel algorithm for non-invasive assessment of fibrosis in NAFLD[J]. PLo S One,2013,8(4):e62439. [29] YIP TC,MA AJ,WONG VW,et al. Laboratory parameterbased machine learning model for excluding non-alcoholic fatty liver disease(NAFLD)in the general population[J]. Aliment Pharmacol Ther,2017,46(4):447-456. [30] FIALOKE S,MALARSTIG A,MILLER MR,et al. Application of machine learning methods to predict non-alcoholic steatohepatitis(NASH)in non-alcoholic fatty liver(NAFL)patients[J]. AMIA Annu Symp Proc,2018,2018:430-439. [31] HAN D,QI XS,YU Y. An excerpt of portal hypertensive bleeding in cirrhosis:Risk stratification,diagnosis and management-2016practice guidance by the American Association for the Study of Liver Diseases[J]. J Clin Hepatol,2017,33(3):422-427.(in Chinese)韩丹,祁兴顺,于洋.《2016年美国肝病学会肝硬化门静脉高压出血的风险分层、诊断和管理实践指导》摘译[J].临床肝胆病杂志,2017,33(3):422-427. [32] HONG WD,JI YF,WANG D,et al. Use of artificial neural network to predict esophageal varices in patients with HBV related cirrhosis[J]. Hepat Mon,2011,11(7):544-547. [33] DONG TS,KALANI A,ABY ES,et al. Machine learningbased development and validation of a scoring system for screening high-risk esophageal varices[J]. Clin Gastroenterol Hepatol,2019,17(9):1894-1901. e1. -
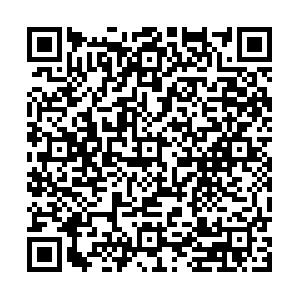
计量
- 文章访问数: 530
- HTML全文浏览量: 29
- PDF下载量: 97
- 被引次数: 0