急性胰腺炎机器学习模型的研究进展
DOI: 10.3969/j.issn.1001-5256.2023.12.034
-
摘要: 急性胰腺炎是一种需要早期干预的消化系统急症,当进展为中度重症或重症急性胰腺炎时,患者病死率显著升高。机器学习凭借强大的计算和学习能力,充分利用临床数据对急性胰腺炎进行早期预测,取得了显著成果。本文综述机器学习在预测急性胰腺炎严重程度、并发症和死亡中的研究进展,为进一步通过人工智能协助急性胰腺炎临床诊疗提供理论依据和新思路。Abstract: Acute pancreatitis (AP) is a gastrointestinal disease that requires early intervention, and when it progresses to moderate-severe AP (MSAP) or severe AP (SAP), there will be a significant increase in the mortality rate of patients. Machine learning (ML) has achieved great success in the early prediction of AP using clinical data with the help of its powerful computational and learning capabilities. This article reviews the research advances in ML in predicting the severity, complications, and death of AP, so as to provide a theoretical basis and new insights for clinical diagnosis and treatment of AP through artificial intelligence.
-
表 1 机器学习在AP中应用研究的基本特点
Table 1. Characteristics of studies on application of machine learning in acute pancreatitis
作者 年份 实验设计 研究目的 样本量 算法 AUC 敏感度/特异度 监督学习 Pearce[13] 2006 回顾性 严重程度 265 核函数-逻辑回归 0.82 0.87/0.71 Sun[14] 2021 回顾性 严重程度 945 随机森林 0.73 0.784/0.618 Langmead[15] 2021 前瞻性 严重程度 60/133 随机森林 0.91 NA Thapa[16] 2021 回顾性 严重程度 61 894 逻辑回归 XGBoost 神经网络 0.780 0.921 0.811 0.850/0.503 0.851/0.830 0.851/0.561 Kui[17] 2022 前瞻性 严重程度 1 184 XGBoost 0.81 NA Yuan[18] 2022 回顾性 严重程度 5 460 XGBoost 线性核函数-支持向量机 径向基核函数-支持向量机 逻辑核函数-支持向量机 逻辑回归 0.952 0.838 0.885 0.862 0.892 NA İnce[19] 2023 回顾性 严重程度 1 334 梯度提升树模型 NA NA Luo[20] 2023 回顾性 严重程度 631/109 随机森林 K近邻算法 朴素贝叶斯 0.961 0.947 0.954 0.900/0.815 0.933/0.667 1.000/0.482 Kiss[22] 2022 前瞻性 严重程度 2 387 XGBoost 0.757 NA Fei[23] 2018 回顾性 并发急性肺损伤 217 神经网络 逻辑回归 0.859±0.048 0.701±0.041 0.875/0.833 0.750/0.708 Xu[8] 2021 回顾性 并发多器官功能衰竭 455 逻辑回归 朴素贝叶斯 支持向量机 AdaBoost 二次判别分析 反向传播网络 0.840 0.864 0.839 0.826 0.865 0.862 0.654/0.900 0.812/0.783 0.713/0.839 0.802/0.804 0.832/0.774 0.832/0.765 Zhang[24] 2023 前瞻性 新发糖尿病 3 477 支持向量机 CatBoost 随机森林 L1-逻辑回归 L2-逻辑回归 神经网络 0.489 0.783 0.788 0.819 0.805 0.751 NA Hameed[25] 2022 回顾性 死亡 6 258 随机森林 决策树 XGBoost 逻辑回归 多层感知器 神经网络 0.942 0.690 0.944 0.875 0.902 0.908 0.273/0.997 0.414/0.967 0.364/0.993 0.141/0.994 0.251/0.992 0.278/0.989 无监督学习 Kimita[26] 2022 前瞻性 识别AP相关细胞因子谱 107 聚类分析 NA NA 注:AUC,受试者工作特征曲线下面积;NA,原文未提供数据。 -
[1] Institute for Health Metrics and Evaluation(IHME). GBD results[EB/OL].[ 2023-10-27]. https://vizhub.healthdata.org/gbd-results/. https://vizhub.healthdata.org/gbd-results/ [2] BANKS PA, BOLLEN TL, DERVENIS C, et al. Classification of acute pancreatitis: 2012: Revision of the Atlanta classification and definitions by international consensus[J]. Gut, 2013, 62( 1): 102- 111. DOI: 10.1136/gutjnl-2012-302779. [3] SINONQUEL P, LALEMAN W, WILMER A. Advances in acute pancreatitis[J]. Curr Opin Crit Care, 2021, 27( 2): 193- 200. DOI: 10.1097/MCC.0000000000000806. [4] WU Q, WANG J, QIN MB, et al. Accuracy of conventional and novel scoring systems in predicting severity and outcomes of acute pancreatitis: A retrospective study[J]. Lipids Health Dis, 2021, 20( 1): 41. DOI: 10.1186/s12944-021-01470-4. [5] BOLLEN TL, SINGH VK, MAURER R, et al. A comparative evaluation of radiologic and clinical scoring systems in the early prediction of severity in acute pancreatitis[J]. Am J Gastroenterol, 2012, 107( 4): 612- 619. DOI: 10.1038/ajg.2011.438. [6] WU BU, BATECH M, QUEZADA M, et al. Dynamic measurement of disease activity in acute pancreatitis: The pancreatitis activity scoring system[J]. Am J Gastroenterol, 2017, 112( 7): 1144- 1152. DOI: 10.1038/ajg.2017.114. [7] HAN TY, CHENG T, LIAO Y, et al. Development and validation of a novel prognostic score based on thrombotic and inflammatory biomarkers for predicting 28-day adverse outcomes in patients with acute pancreatitis[J]. J Inflamm Res, 2022, 15: 395- 408. DOI: 10.2147/JIR.S344446. [8] XU FM, CHEN X, LI C, et al. Prediction of multiple organ failure complicated by moderately severe or severe acute pancreatitis based on machine learning: A multicenter cohort study[J]. Mediators Inflamm, 2021, 2021: 5525118. DOI: 10.1155/2021/5525118. [9] YE JF, ZHAO YX, JU J, et al. Building and verifying a severity prediction model of acute pancreatitis(AP) based on BISAP, MEWS and routine test indexes[J]. Clin Res Hepatol Gastroenterol, 2017, 41( 5): 585- 591. DOI: 10.1016/j.clinre.2016.11.013. [10] ZHAO B, SUN SL, WANG YH, et al. Cardiac indicator CK-MB might be a predictive marker for severity and organ failure development of acute pancreatitis[J]. Ann Transl Med, 2021, 9( 5): 368. DOI: 10.21037/atm-20-3095. [11] DEO RC. Machine learning in medicine[J]. Circulation, 2015, 132( 20): 1920- 1930. DOI: 10.1161/CIRCULATIONAHA.115.001593. [12] JIN QW, FU LJ, YANG HX, et al. Peripheral lymphocyte count defines the clinical phenotypes and prognosis in patients with anti-MDA5-positive dermatomyositis[J]. J Intern Med, 2023, 293( 4): 494- 507. DOI: 10.1111/joim.13607. [13] PEARCE CB, GUNN SR, AHMED A, et al. Machine learning can improve prediction of severity in acute pancreatitis using admission values of APACHE II score and C-reactive protein[J]. Pancreatology, 2006, 6( 1-2): 123- 131. DOI: 10.1159/000090032. [14] SUN HW, LU JY, WENG YX, et al. Accurate prediction of acute pancreatitis severity with integrative blood molecular measurements[J]. Aging, 2021, 13( 6): 8817- 8834. DOI: 10.18632/aging.202689. [15] LANGMEAD C, LEE PJ, PARAGOMI P, et al. A novel 5-cytokine panel outperforms conventional predictive markers of persistent organ failure in acute pancreatitis[J]. Clin Transl Gastroenterol, 2021, 12( 5): e00351. DOI: 10.14309/ctg.0000000000000351. [16] THAPA R, IQBAL Z, GARIKIPATI A, et al. Early prediction of severe acute pancreatitis using machine learning[J]. Pancreatology, 2022, 22( 1): 43- 50. DOI: 10.1016/j.pan.2021.10.003. [17] KUI B, PINTÉR J, MOLONTAY R, et al. EASY-APP: An artificial intelligence model and application for early and easy prediction of severity in acute pancreatitis[J]. Clin Transl Med, 2022, 12( 6): e842. DOI: 10.1002/ctm2.842. [18] YUAN L, JI MY, WANG S, et al. Machine learning model identifies aggressive acute pancreatitis within 48 h of admission: A large retrospective study[J]. BMC Med Inform Decis Mak, 2022, 22( 1): 312. DOI: 10.1186/s12911-022-02066-3. [19] İNCE AT, SILAHTAROĞLU G, SEVEN G, et al. Early prediction of the severe course, survival, and ICU requirements in acute pancreatitis by artificial intelligence[J]. Pancreatology, 2023, 23( 2): 176- 186. DOI: 10.1016/j.pan.2022.12.005. [20] LUO Z, SHI JL, FANG YY, et al. Development and evaluation of machine learning models and nomogram for the prediction of severe acute pancreatitis[J]. J Gastroenterol Hepatol, 2023, 38( 3): 468- 475. DOI: 10.1111/jgh.16125. [21] BUGIANTELLA W, RONDELLI F, BONI M, et al. Necrotizing pancreatitis: A review of the interventions[J]. Int J Surg, 2016, 28( Suppl 1): S163- S171. DOI: 10.1016/j.ijsu.2015.12.038. [22] KISS S, PINTÉR J, MOLONTAY R, et al. Early prediction of acute necrotizing pancreatitis by artificial intelligence: A prospective cohort-analysis of 2387 cases[J]. Sci Rep, 2022, 12( 1): 7827. DOI: 10.1038/s41598-022-11517-w. [23] FEI Y, GAO K, LI WQ. Artificial neural network algorithm model as powerful tool to predict acute lung injury following to severe acute pancreatitis[J]. Pancreatology, 2018, 18( 8): 892- 899. DOI: 10.1016/j.pan.2018.09.007. [24] ZHANG J, LV YQ, HOU JY, et al. Machine learning for post-acute pancreatitis diabetes mellitus prediction and personalized treatment recommendations[J]. Sci Rep, 2023, 13( 1): 4857. DOI: 10.1038/s41598-023-31947-4. [25] HAMEED MAB, ALAMGIR Z. Improving mortality prediction in acute pancreatitis by machine learning and data augmentation[J]. Comput Biol Med, 2022, 150: 106077. DOI: 10.1016/j.compbiomed.2022.106077. [26] KIMITA W, BHARMAL SH, KO J, et al. Identifying endotypes of individuals after an attack of pancreatitis based on unsupervised machine learning of multiplex cytokine profiles[J]. Transl Res, 2023, 251: 54- 62. DOI: 10.1016/j.trsl.2022.07.001. [27] YIN MY, LIANG XL, WANG ZL, et al. Identification of asymptomatic COVID-19 patients on chest CT images using transformer-based or convolutional neural network-based deep learning models[J]. J Digit Imaging, 2023, 36( 3): 827- 836. DOI: 10.1007/s10278-022-00754-0. [28] COGAN T, COGAN M, TAMIL L. MAPGI: Accurate identification of anatomical landmarks and diseased tissue in gastrointestinal tract using deep learning[J]. Comput Biol Med, 2019, 111: 103351. DOI: 10.1016/j.compbiomed.2019.103351. [29] CHO BJ, BANG CS, PARK SW, et al. Automated classification of gastric neoplasms in endoscopic images using a convolutional neural network[J]. Endoscopy, 2019, 51( 12): 1121- 1129. DOI: 10.1055/a-0981-6133. [30] TONOZUKA R, ITOI T, NAGATA N, et al. Deep learning analysis for the detection of pancreatic cancer on endosonographic images: A pilot study[J]. J Hepatobiliary Pancreat Sci, 2021, 28( 1): 95- 104. DOI: 10.1002/jhbp.825. [31] DURAK S, BAYRAM B, BAKIRMAN T, et al. Deep neural network approaches for detecting gastric polyps in endoscopic images[J]. Med Biol Eng Comput, 2021, 59( 7-8): 1563- 1574. DOI: 10.1007/s11517-021-02398-8. [32] de GROOF AJ, STRUYVENBERG MR, FOCKENS KN, et al. Deep learning algorithm detection of Barrett's neoplasia with high accuracy during live endoscopic procedures: A pilot study(with video)[J]. Gastrointest Endosc, 2020, 91( 6): 1242- 1250. DOI: 10.1016/j.gie.2019.12.048. [33] LIU YZ, LEI Y, FU YB, et al. CT-based multi-organ segmentation using a 3D self-attention U-net network for pancreatic radiotherapy[J]. Med Phys, 2020, 47( 9): 4316- 4324. DOI: 10.1002/mp.14386. [34] AN P, YANG DM, WANG J, et al. A deep learning method for delineating early gastric cancer resection margin under chromoendoscopy and white light endoscopy[J]. Gastric Cancer, 2020, 23( 5): 884- 892. DOI: 10.1007/s10120-020-01071-7. [35] FENG RW, LIU XC, CHEN JT, et al. A deep learning approach for colonoscopy pathology WSI analysis: Accurate segmentation and classification[J]. IEEE J Biomed Health Inform, 2021, 25( 10): 3700- 3708. DOI: 10.1109/JBHI.2020.3040269. [36] SCHWENDICKE F, GOLLA T, DREHER M, et al. Convolutional neural networks for dental image diagnostics: A scoping review[J]. J Dent, 2019, 91: 103226. DOI: 10.1016/j.jdent.2019.103226. [37] TONG T, GU JH, XU D, et al. Deep learning radiomics based on contrast-enhanced ultrasound images for assisted diagnosis of pancreatic ductal adenocarcinoma and chronic pancreatitis[J]. BMC Med, 2022, 20( 1): 74. DOI: 10.1186/s12916-022-02258-8. [38] CHEN PT, CHANG DW, WU TH, et al. Applications of artificial intelligence in pancreatic and biliary diseases[J]. J Gastroenterol Hepatol, 2021, 36( 2): 286- 294. DOI: 10.1111/jgh.15380. [39] HUANG SC, PAREEK A, SEYYEDI S, et al. Fusion of medical imaging and electronic health records using deep learning: A systematic review and implementation guidelines[J]. NPJ Digit Med, 2020, 3: 136. DOI: 10.1038/s41746-020-00341-z. [40] LV ZL, LIN YX, YAN R, et al. TransSurv: Transformer-based survival analysis model integrating histopathological images and genomic data for colorectal cancer[J]. IEEE/ACM Trans Comput Biol Bioinform, 2022. DOI: 10.1109/TCBB.2022.3199244.[ Online ahead of print] [41] SOENKSEN LR, MA Y, ZENG C, et al. Integrated multimodal artificial intelligence framework for healthcare applications[J]. NPJ Digit Med, 2022, 5( 1): 149. DOI: 10.1038/s41746-022-00689-4. [42] WANG RJ, DAI WX, GONG J, et al. Development of a novel combined nomogram model integrating deep learning-pathomics, radiomics and immunoscore to predict postoperative outcome of colorectal cancer lung metastasis patients[J]. J Hematol Oncol, 2022, 15( 1): 11. DOI: 10.1186/s13045-022-01225-3. [43] WANG Y, HONG Y, WANG Y, et al. Automated multimodal machine learning for esophageal variceal bleeding prediction based on endoscopy and structured data[J]. J Digit Imaging, 2023, 36( 1): 326- 338. DOI: 10.1007/s10278-022-00724-6. [44] WANG LH, YAN DF, SHEN L, et al. Prognostic value of a CT radiomics-based nomogram for the overall survival of patients with nonmetastatic BCLC stage C hepatocellular carcinoma after stereotactic body radiotherapy[J]. J Oncol, 2023, 2023: 1554599. DOI: 10.1155/2023/1554599. [45] HUANG JZ, XIE XH, WU H, et al. Development and validation of a combined nomogram model based on deep learning contrast-enhanced ultrasound and clinical factors to predict preoperative aggressiveness in pancreatic neuroendocrine neoplasms[J]. Eur Radiol, 2022, 32( 11): 7965- 7975. DOI: 10.1007/s00330-022-08703-9. -
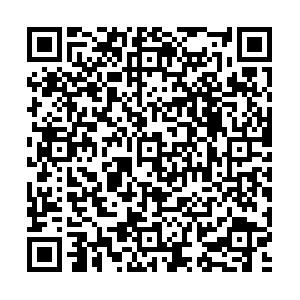
计量
- 文章访问数: 296
- HTML全文浏览量: 222
- PDF下载量: 58
- 被引次数: 0